AI: Coming to a smart building near you?
作者
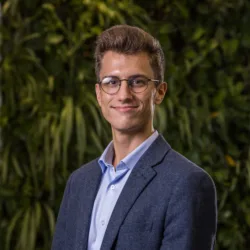
Laszlo Karsai
查看个人简介The explosion of ChatGPT, Stable Diffusion, Google Bard and others into the mainstream in the last two years has shown that artificial intelligence (AI) will have real impacts across every sector. Generative AI has proven capable of responding to questions, creating new images, debugging code, and much more. But what about its use in the built environment? Let’s explore what AI means for smart buildings in particular.
AI presents a great opportunity for the decarbonisation of buildings via the optimisation of building controls. For example, heating, ventilation and air conditioning (HVAC) systems account for about 40% of a building’s energy consumption, meaning HVAC optimisations can create considerable cost savings and carbon savings over time. Ideally, HVAC controls should work to balance the occupancy of the office versus outdoor air conditions. However, in practice, systems often run on a timer with minor seasonal setpoint changes. With AI, these HVAC controls can be automatically enabling the system to make changes over time.
Dynamic learning is a key benefit of AI tools over existing smart platforms. Landsec, the commercial property developer and London’s largest office owner is trialling a platform called Brainbox AI to reduce their carbon emissions via the tuning of building systems. The AI platform is ‘connected’ to the building and ingests data over one year, learning how the building is used day-to-day and suggesting tweaks to systems based on indoor air quality, occupancy, grid energy rates, or weather. The more the AI learns, the more accurately it can tune systems to generate savings. A Montreal office building using Brainbox AI has reported 11% electricity savings on HVAC equipment in the first five months of operation, including equipment runtime reductions exceeding 30%.
Many efficiency gains are to be made in the process of optimising controls. A typical manual workflow can be summarised as follows:
- An issue is detected/raised.
- Troubleshooting/data analysis to understand the problem takes place.
- A new setpoint is tested.
- The facilities management (FM) team implements the change.
If AI can be entrusted with on-the-fly optimisation, timing and setpoint changes are handled automatically. Facility management FM teams will simply receive a notification of the action and results.
Hank, another AI-powered optimisation platform, took control of an American commercial office, making 200 adjustments per hour, resulting in 31% HVAC energy savings and three fewer service callouts monthly. Not only does this reduce the labour effort of manual control optimisation, but it also allows issues (such as a faulty mechanical plant) to be flagged before they cause downtime. This means FM teams can be more flexibly resourced across multiple buildings, improving service efficiency across the portfolio. Additionally, tools with chatbot interfaces – familiar to those who’ve used ChatGPT – will allow FM teams and asset managers to query their building data conversationally: “How many kWh of energy was used by lighting whilst the office was closed?” or “Show me when the AHU filter has been replaced in the last five years.” This will be a bonus for property owners who do not have data analysts at their disposal.
For large landlords and developers, the ability to analyse datasets across their portfolios will become ever more important. JLL’s JLL-GPT tool, an AI model developed specifically for commercial real estate, “uses market data, external business insights and internal commercial real estate data to generate insights for decision-making”. Assets that are underperforming can be flagged, and improvements suggested – whether that is maintenance, retrofit, or selling. Compliance against sustainability targets or other accreditations could also be tracked dynamically. Once all buildings in a portfolio feed data into the platform, AI will simplify truly data-driven portfolio management, making it easier for developers to see the benefits of decarbonisation.
If smart buildings (especially AI-powered ones) are so valuable, why aren’t all new builds going smart? Smart buildings have been around for a while. Still, widespread adoption has been slow, with varying definitions, poor understanding in the industry, the high operational expenditure (OPEX) of smart platforms, and uncertainty over return on investment. Reliable data proving the decarbonisation potential of smart buildings will be vital in changing attitudes, and as sustainability targets become more ambitious, smart solutions will play a central role in helping to meet them. The financial benefits of going smart should, in turn, crystallise through the reduced cost of upkeep, higher rent premiums and the ability to avoid the asset stranding of buildings 15-30 years down the line.
Retrofit is an even greater challenge, given that 80% of the buildings projected to be in use in 2050 have already been built. Legacy buildings often feature siloed HVAC systems and inadequate IT infrastructure – these will require a targeted approach with select system upgrades and quick-to-deploy software, unlocking the building’s data and enabling AI optimisation. Smart buildings are crucial to decarbonising the built environment, so let’s accelerate the building technology transition and embrace AI.